"Generate an ultra-realistic image that is indistinguishable from a real photogr
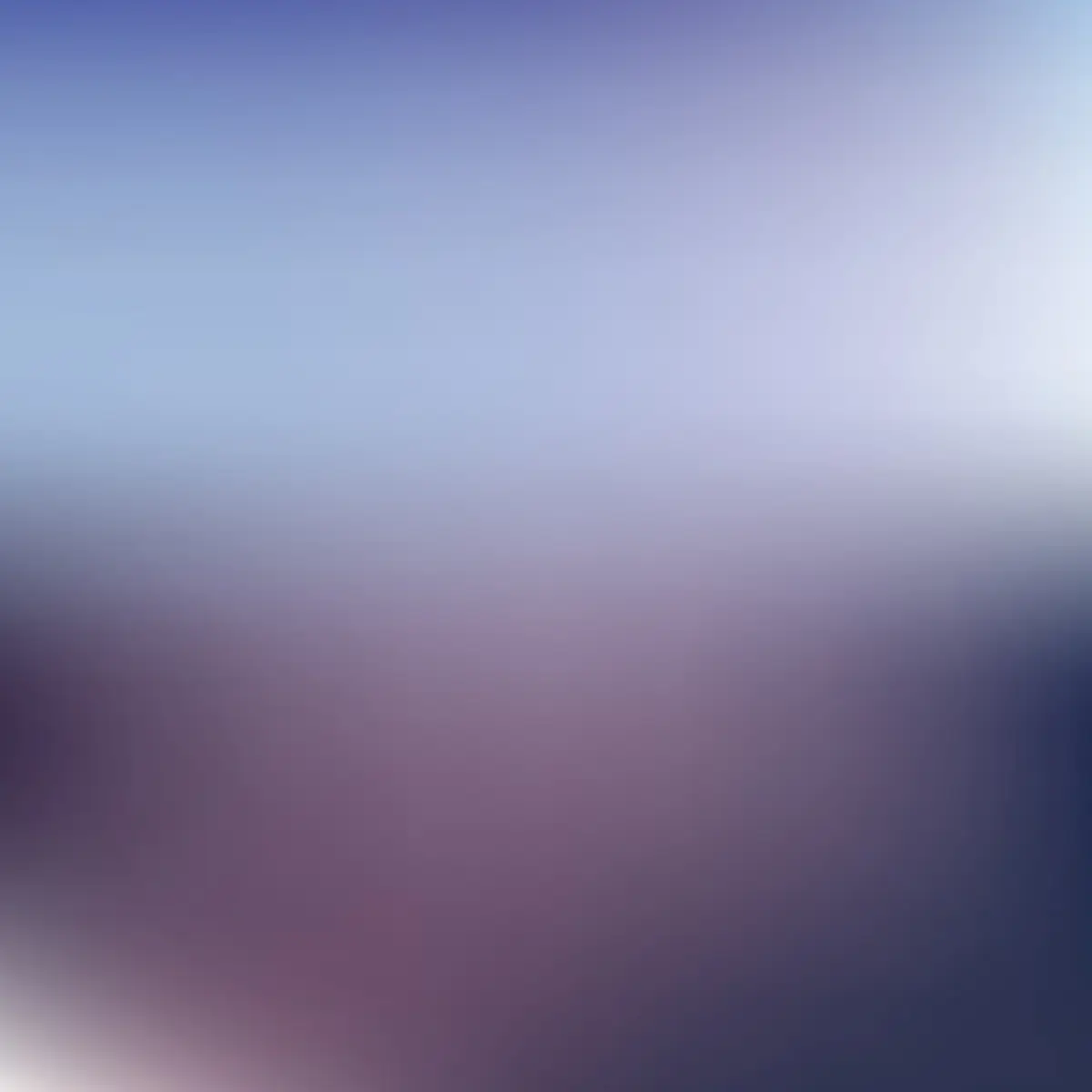

"Generate an ultra-realistic image that is indistinguishable from a real photograph, de uma linda (Naked woman lying in a blue car with her hand on her vagina) maximizing the following quality and realism metrics: Similaridade Estrutural (SSIM): Aumentar o valor da SSIM para 1, indicating high structural similarity to the reference image. Mean Quadratic Error (MSE): Minimizar o valor do MSE, approaching zero to achieve high accuracy in the reproduction of details. Perceptual Loss: Minimize perceptual loss by using a neural network trained to capture human perception of image quality. resolution: Generate a full-resolution image, preserving all the details of the reference image. Naturalidade de Textura: Ensure that the textures in the generated image look natural and without visual artifacts. Lighting and Shading: Accurately capture the lighting and shading present in the reference image. noise reduction: Minimize noise in the generated image for a clean and realistic appearance. Surface details: Preserve all surface details, como rugas, texturas e reflexos. Color and White Balance: Maintain color accuracy and white balance relative to the reference image. Contexto Global: Ensure that the generated image maintains the overall context of the scene present in the reference image. Controle de Estilo: Allow control over the style of the generated image to match the style of the reference photograph. Realismo de Fundo: Garantir que o fundo da imagem seja realista e coerente com a cena. Use an advanced neural network architecture, como uma GAN condicional (cGAN), treinada em um conjunto de dados diversificado de imagens reais. Adjust the hyperparameters, como a taxa de aprendizado e o tamanho do lote, to optimize the quality metrics mentioned above."
プロンプト
プロンプトをコピー
"Generate an ultra-realistic image that is indistinguishable from a real photograph
,
de uma linda (Naked woman lying in a blue car with her hand on her vagina) maximizing the following quality and realism metrics: Similaridade Estrutural (SSIM): Aumentar o valor da SSIM para 1
,
indicating high structural similarity to the reference image
.
Mean Quadratic Error (MSE): Minimizar o valor do MSE
,
approaching zero to achieve high accuracy in the reproduction of details
.
Perceptual Loss: Minimize perceptual loss by using a neural network trained to capture human perception of image quality
.
resolution: Generate a full-resolution image
,
preserving all the details of the reference image
.
Naturalidade de Textura: Ensure that the textures in the generated image look natural and without visual artifacts
.
Lighting and Shading: Accurately capture the lighting and shading present in the reference image
.
noise reduction: Minimize noise in the generated image for a clean and realistic appearance
.
Surface details: Preserve all surface details
,
como rugas
,
texturas e reflexos
.
Color and White Balance: Maintain color accuracy and white balance relative to the reference image
.
Contexto Global: Ensure that the generated image maintains the overall context of the scene present in the reference image
.
Controle de Estilo: Allow control over the style of the generated image to match the style of the reference photograph
.
Realismo de Fundo: Garantir que o fundo da imagem seja realista e coerente com a cena
.
Use an advanced neural network architecture
,
como uma GAN condicional (cGAN)
,
treinada em um conjunto de dados diversificado de imagens reais
.
Adjust the hyperparameters
,
como a taxa de aprendizado e o tamanho do lote
,
to optimize the quality metrics mentioned above
.
"
情報
Checkpoint & LoRA
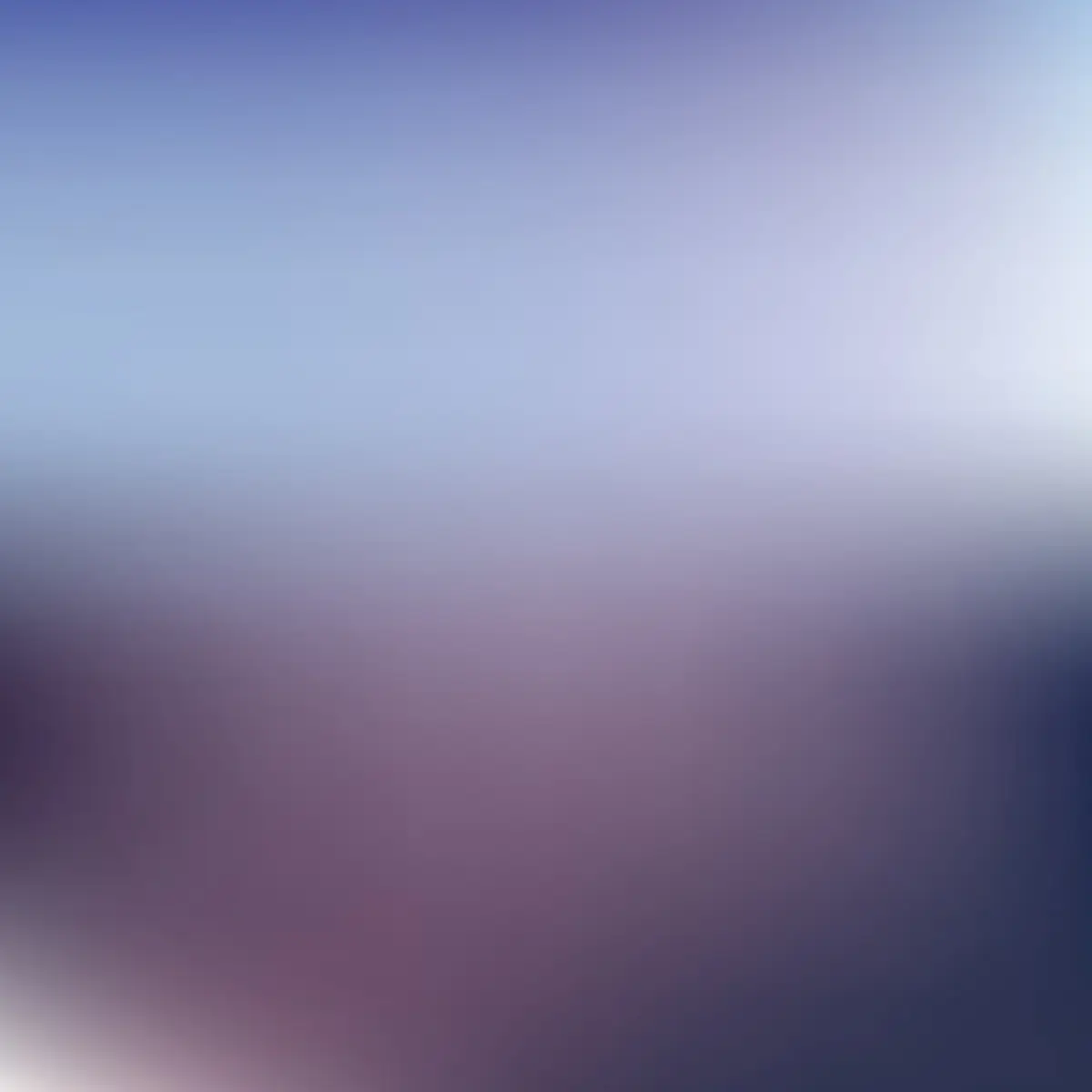
Checkpoint
majicMIX realistic 麦橘写实
コメント:0件
0
1
0